
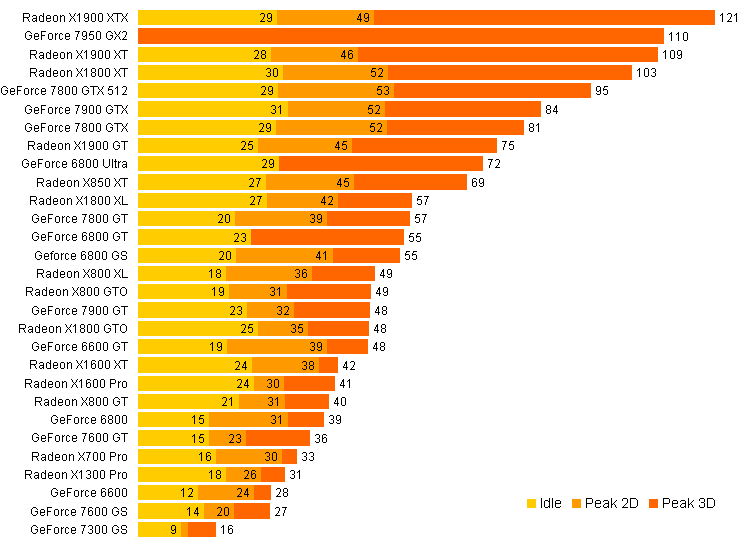
This is also called the latency of the operation. Thus we essentially have a queue where the next operations needs to wait for the next operation to finish. However, most of the time, operations take longer than one cycle. Each cycle represents an opportunity for computation. If a processor runs at 1GHz, it can do 10^9 cycles per second. To understand this example fully, you have to understand the concepts of cycles. A CUDA programmer would take this as a first “draft” and then optimize it step-by-step with concepts like double buffering, register optimization, occupancy optimization, instruction-level parallelism, and many others, which I will not discuss at this point. This is a simplified example, and not the exact way how a high performing matrix multiplication kernel would be written, but it has all the basics. Here I will show you a simple example of A*B=C matrix multiplication, where all matrices have a size of 32×32, what a computational pattern looks like with and without Tensor Cores. It is helpful to understand how they work to appreciate the importance of these computational units specialized for matrix multiplication. In fast, they are so powerful, that I do not recommend any GPUs that do not have Tensor Cores. Since the most expensive part of any deep neural network is matrix multiplication Tensor Cores are very useful. Tensor Cores are tiny cores that perform very efficient matrix multiplication. Tensor Cores are most important, followed by memory bandwidth of a GPU, the cache hierachy, and only then FLOPS of a GPU. This section is sorted by the importance of each component. This understanding will help you to evaluate future GPUs by yourself.
#Compare graphics cards models how to#
This section can help you build a more intuitive understanding of how to think about deep learning performance. The Most Important GPU Specs for Deep Learning Processing Speed If we look at the details, we can understand what makes one GPU better than another. This is a high-level explanation that explains quite well why GPUs are better than CPUs for deep learning. The best high-level explanation for the question of how GPUs work is my following Quora answer: Read Tim Dettmers‘ answer to Why are GPUs well-suited to deep learning? on Quora You can skip this section if you just want the useful performance numbers and arguments to help you decide which GPU to buy. In turn, you might be able to understand better why you need a GPU in the first place and how other future hardware options might be able to compete. This knowledge will help you to undstand cases where are GPUs fast or slow. If you use GPUs frequently, it is useful to understand how they work. After that follows a Q&A section of common questions posed to me in Twitter threads in that section, I will also address common misconceptions and some miscellaneous issues, such as cloud vs desktop, cooling, AMD vs NVIDIA, and others. From there, I make GPU recommendations for different scenarios.
#Compare graphics cards models series#
I discuss the unique features of the new NVIDIA RTX 40 Ampere GPU series that are worth considering if you buy a GPU. These explanations might help you get a more intuitive sense of what to look for in a GPU. I will discuss CPUs vs GPUs, Tensor Cores, memory bandwidth, and the memory hierarchy of GPUs and how these relate to deep learning performance. First, I will explain what makes a GPU fast. This blog post is structured in the following way. You might want to skip a section or two based on your understanding of the presented topics. (3) If you want to get an in-depth understanding of how GPUs, caches, and Tensor Cores work, the best is to read the blog post from start to finish. (2) If you worry about specific questions, I have answered and addressed the most common questions and misconceptions in the later part of the blog post. The cost/performance numbers form the core of the blog post and the content surrounding it explains the details of what makes up GPU performance.
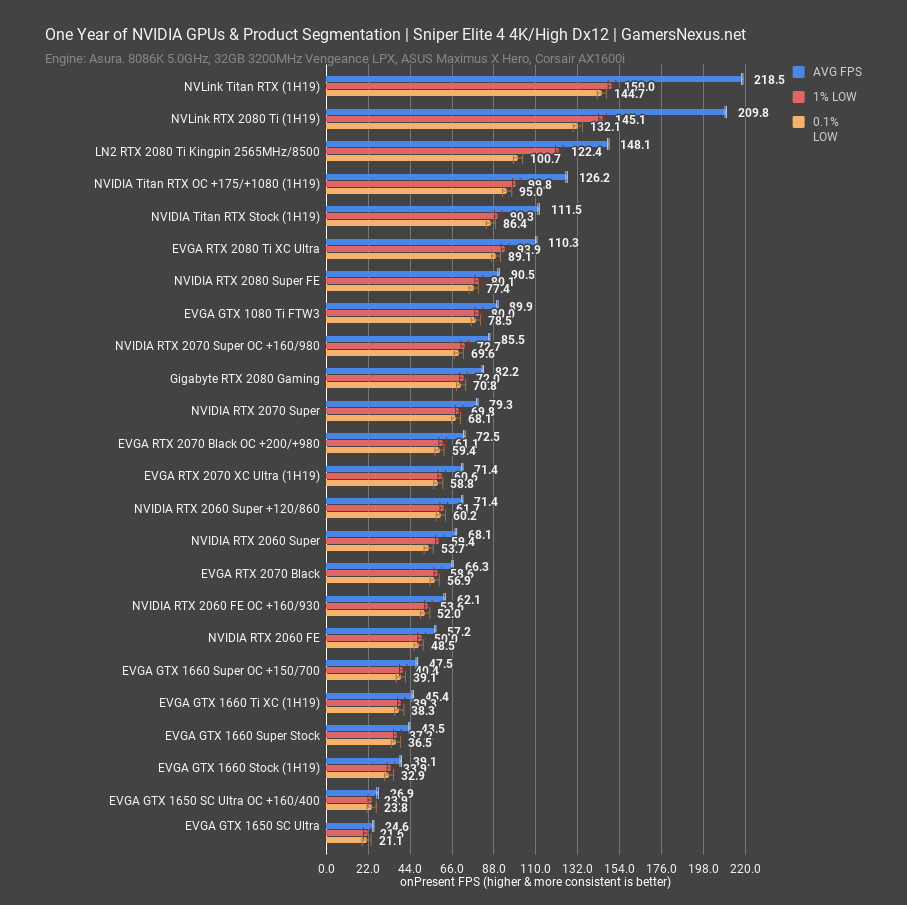
You have the choice: (1) If you are not interested in the details of how GPUs work, what makes a GPU fast compared to a CPU, and what is unique about the new NVIDIA RTX 40 Ampere series, you can skip right to the performance and performance per dollar charts and the recommendation section. This blog post is designed to give you different levels of understanding of GPUs and the new Ampere series GPUs from NVIDIA. But what features are important if you want to buy a new GPU? GPU RAM, cores, tensor cores, caches? How to make a cost-efficient choice? This blog post will delve into these questions, tackle common misconceptions, give you an intuitive understanding of how to think about GPUs, and will lend you advice, which will help you to make a choice that is right for you. Deep learning is a field with intense computational requirements, and your choice of GPU will fundamentally determine your deep learning experience.
